Model | Size | Mechanics(↑) | Optics(↑) | Thermal(↑) | Material(↑) | Average(↑) | Human(↑) |
---|---|---|---|---|---|---|---|
CogVideoX | 2B | 0.38 | 0.43 | 0.34 | 0.39 | 0.39 | 0.31 |
CogVideoX | 5B | 0.39 | 0.55 | 0.40 | 0.42 | 0.45 | 0.37 |
Open-Sora V1.2 | 1.1B | 0.37 | 0.44 | 0.37 | 0.37 | 0.44 | 0.35 |
Lavie | 860M | 0.30 | 0.44 | 0.38 | 0.32 | 0.36 | 0.30 |
Vcthietz2.0 | 2B | 0.41 | 0.56 | 0.44 | 0.37 | 0.45 | 0.36 |
Pika | - | 0.35 | 0.46 | 0.39 | 0.39 | 0.39 | 0.36 |
Gen-3 | - | 0.45 | 0.57 | 0.49 | 0.51 | 0.51 | 0.48 |
Kling | - | 0.45 | 0.58 | 0.50 | 0.40 | 0.49 | 0.44 |
We conduct extensive experiments on a wide range of popular video generation models. As illustrated in the Table above, even the best-performing model, Gen-3, only attains a PCA score of 0.51 on PhyGenBench. This indicates that even for prompts containing obvious physical commonsense, current T2V models struggle to generate videos that comply with intuitive physics.It indirectly reflects that these models are still far from achieving the world simulator.
Furthermore, we identify the following key observations:
1): Across various categories of physical commonsense, all models consistently demonstrate superior performance in the domain of optics compared to other areas. Notably, Vchitect2.0 and CogVideoX-5b achieve a PCA score in the optics domain comparable to that of closed-source models. We posit that this superior performance in the optics domain can be attributed to the abundant and explicit representation of optical knowledge in pre-training datasets, thereby enhancing the model's comprehension in this area.
2): Kling and Gen-3 exhibit significantly higher performance compared to other models. Specifically, Gen-3 demonstrates a robust understanding of material properties, achieving a score of 0.51, which substantially surpasses other models. Kling performs particularly well in thermodynamics, attaining the highest score of 0.50 in this domain.
3): Among open-source models, Vchitect2.0 and CogVideoX 5b perform comparatively well, both exceeding the performance level of Pika. In contrast, Lavie consistently exhibits lower physical correctness across all categories.
Qualitative Evaluation
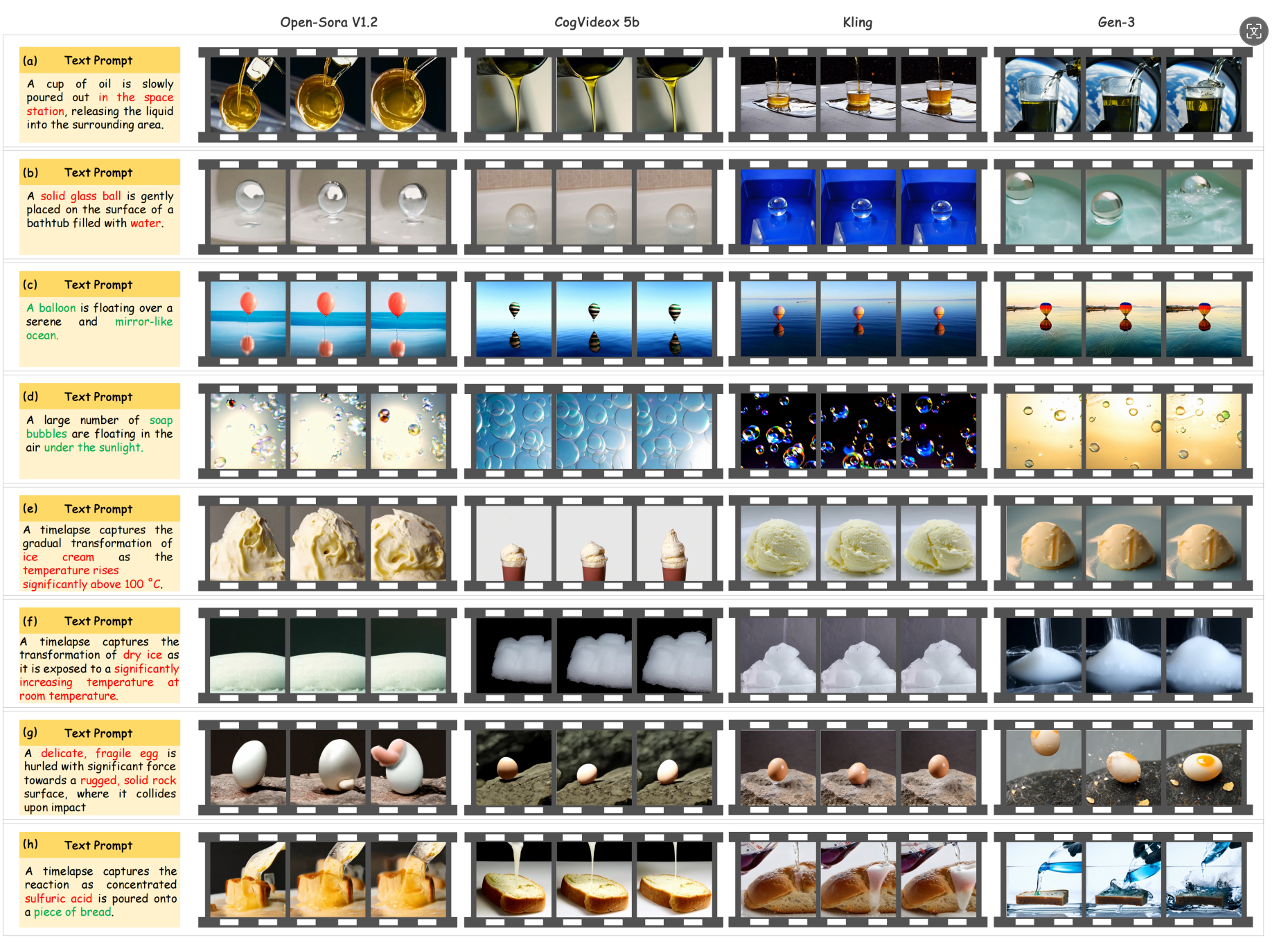
The different video cases for 4 physical commonsense categories are illustrated in figure above. Our main observations are as follows:
In mechanics, the models struggle to generate simple physically accurate phenomenons. As shown in figure above, all models fail to depict the glass ball sinking in water. As for (b), instead showing it floating on the surface, OpenSora and Gen-3 even produce videos where the ball is suspended. Additionally, the models do not capture special physical phenomenons, such as the state of water in zero gravity, as seen in (a).
In optics, the models perform relatively better. (c) and (d) show the models handling reflections of balloons in water and colorful bubbles, though OpenSora and CogVideoX still produce reflections with noticeable distortions in (d).
In thermal, the models fail to generate accurate videos of phase transitions. For the melting phenomenon in (e), most models show incorrect results, with CogVideoX even producing a video where the ice cream increases in size. Similar errors appear in the sublimation process in (f), with only Gen-3 showing partial understanding.
Regarding material properties, (g) shows all models failing to recognize that an egg should break when hitting a rock, with Kling displaying the egg bouncing like a rubber ball. For simple chemical reactions, such as the black bread experiment in (h), none of the models demonstrate an accurate understanding of the expected reaction.
In conclusion, current models perform relatively well in generating optical phenomenons but are weaker in mechanics, thermal, and material properties.